Transforming Supply Chain Due Diligence: From Funnel to Hourglass with AI
How AI and GenAI Are Revolutionizing Supplier Risk Management by Enhancing Transparency, Efficiency, and Scalability
by Craig Moss and Dave Ferrucci
Your company has 10,000 suppliers in 30 countries. You may be concerned about the potential use of forced labor in your supply chain or struggling to track suppliers’ carbon emissions. Customers are demanding better supply chain due diligence to mitigate their own social and environmental risks. In some countries, regulations now require you to not only assess your suppliers’ environmental and social risks but also take action to reduce them—and publicly report your efforts.
Historically, companies have relied on a breadth-and-depth approach: conduct a broad assessment of all 10,000 suppliers to determine their inherent risk based on factors like location and the nature of their business, then narrow the focus to 100-200 key suppliers for deeper analysis. These suppliers are typically selected based on how critical they are, the amount of money spent with them, and their inherent risk. However, this model is not scalable and relies too heavily on inherent risk assumptions.
A New Approach: Using AI and GenAI to Build a Scalable Model
By combining deep expertise in supply chain risk management with the application of artificial intelligence (AI) and more specifically, generative artificial intelligence (Gen AI), a new scalable approach has emerged. Instead of relying solely on broad assessments, this approach focuses on residual risk—the risk that remains after accounting for a supplier’s risk management capabilities. This new approach turns a funnel into an hourglass by using the residual risk data from the 100 to extrapolate across the 10,000 suppliers.
Here is how the model works:
- Select the 100 from the 10,000. This can be done using the today’s common techniques by assessing criticality, spend, and inherent risk. In the future, the quality of this selection process will be improved by the results from the bottom of the hourglass.
- Assess the program maturity and residual risk of the 100 suppliers. Gain visibility into the systems and controls that these suppliers use to manage environmental and social risk. Knowing the maturity of your suppliers’ management systems is essential to knowing the residual risk they pose. This will take you far beyond generalizations that suppliers in one country are riskier than those in another country. Gaining visibility into the residual risk also allows you to be far more effective in allocating resources and prioritizing where to focus.
- Extrapolate residual risk to the 10,000 suppliers. Using AI/GenAI, the insights gathered from the 100 can be scaled back up to better understand the overall residual risk of your complete supply chain. Suddenly, you can go from the 10,000 to the 100 and back to the 10,000. This turns the traditional funnel into an hourglass, allowing companies to move between granular and broad risk assessments efficiently.
AI-Powered Risk Prediction and Transparency
Although AI/Gen AI can be used to select the 100 from the 10,000 at the top of the hourglass, we are going to focus on the bottom of the hourglass. The supply chain maturity assessment data (and resulting residual risk data) gathered from the 100 can be used with AI/Gen AI to find patterns and distinguish those patterns based on a wealth of conditional context. So, when you are looking to apply what you learned from the assessments in the funnel to the broader data set (that is, the bottom of the hourglass) understanding how bigger data clusters and groups behave from a risk perspective gives you predictive and explanatory power. In effect, you are able to say which of your suppliers is likely to have high residual risk because of how similar they are to other high-risk suppliers, and different they are from lower risk suppliers, according to various criteria.
Trust and transparency are critical to supply chain risk management and to the use of AI and Gen AI. In both cases, trust and transparency start with the data. Understanding the reliability, currency, and consistency of the data sources used to train the AI is essential. The saying “garbage in, garbage out” has never been more accurate. For example, using a supply chain due diligence maturity assessment designed by experts provides valuable data on the program maturity of your suppliers that goes well beyond asking simple yes/no questions. This helps address the challenge with GenAI where the precise sources used to generate an answer are not readily available from the underlying Large Language Model (LLM). The data generated from the supply chain due diligence maturity assessment is combined in the LLM with additional public data and your internal proprietary data to gather nuanced insights.
Additionally, using Retrieval Augmented Generation (RAG) systems that combine LLMs with search can ensure that AI responses are backed by verifiable sources. This involves pinpointing the original data sources, narrowing the scope of data from which answers are generated, and providing direct access to those sources, including a reliability score. RAG solutions can increase the transparency related to the precise data contributed to those answers linking to original sources. To further improve transparency, we can ensure the process revealed by the LLM for summarizing that data follow established rules for helping to ensure trust.
AI models sometimes generate inaccurate or misleading results called hallucinations. This problem can be reduced through the utilization of reliable residual risk data from the narrow part of the hourglass. The power of GenAI is its ability to predict answers based on patterns in the data. The predictions are heavily conditioned by the context in the query, which means the resulting answer is a probabilistic prediction. Using the residual risk data is one key factor in improving the reliability of the prediction.
A Use Case: AI-Driven Supply Chain Risk Management
Imagine an assessment reveals that most of your top 100 suppliers have high residual risk due to weak social compliance policies and inadequate training. GenAI can:
- Identify patterns in high-risk suppliers. AI detects which supplier characteristics are most associated with high risk. These patterns are then applied to the entire supplier base, allowing companies to predict which of the 10,000 suppliers are likely to pose similar risks Moreover, reinforcement learning (correcting where the AI made a mistake in forming and applying the pattern) allows for quick and iterative improvement to the assessment of the residual risk in a transparent way.
- Generate tailored supplier communications. Following this example, we can combine the data showing a weakness in social policies and training among your suppliers with information on new regulations requiring enhanced due diligence to uncover forced labor. This new regulation requires your company update its Supplier Code of Conduct and communicate it to all suppliers and internally to those that manage the suppliers. The challenge is you have is customizing communications to 10,000 suppliers in 30 different national legal jurisdictions, and which span a variety of business types (e.g., contract manufacturers, logistics companies, employment agencies.) GenAI can modify your Supplier Code of Conduct based on the law and your increased requirement that suppliers have strong internal policies and conduct internal training on forced labor. AI can draft a customized communication for each supplier based on the nature of their business, their country, and any unique conditions imposed by their country. This will dramatically reduce the time to respond and quite likely improve the quality of the result.
- Enhance internal training and compliance. Gen AI can analyze which of your internal teams interact with the highest risk suppliers and tailor training and communication materials that use the specific forced labor scenarios they are most likely to encounter.
Many companies are using or experimenting with AI/Gen AI, but few have successfully integrated it into a scalable supply chain due diligence program. The solution is to turn the funnel into an hourglass. By assessing program maturity of a subset of your suppliers and determining their residual risk, AI/GenAI will enable you to gain visibility into the broader environmental and social residual risk of your entire supply chain while taking reasonable steps to build a scalable program that better meets the requirements of customers, investors, and regulators.
RELATED MATERIAL
How AI Helps Key Partners Manage Supply Chain Risk
How AI Can Upscale Your Supply Chain Risk Management
ABOUT THE AUTHORS
Craig Moss is Executive Vice President of Measurement at Ethisphere and a leading expert on using management systems to improve compliance and risk management performance within companies and across supply chains. He is also a Director at the Digital Supply Chain Institute, where he developed a program to accelerate and scale digital transformation, and a unique new data trading framework.
Dave Ferrucci is an award-winning Artificial Intelligence researcher and keynote speaker who created IBM’s Watson and led the Watson team from its inception in 2006 to its celebrated success in 2011 when Watson defeated the greatest Jeopardy players of all time. Since then, Dave has helped companies within the financial services and healthcare industries implement AI in their own operations. He is presently Managing Director for the Institute for Advanced Enterprise AI.
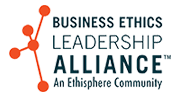
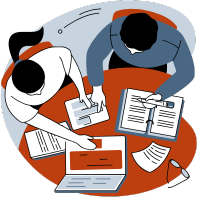